Hard-wired visual filters for environment-agnostic object recognition
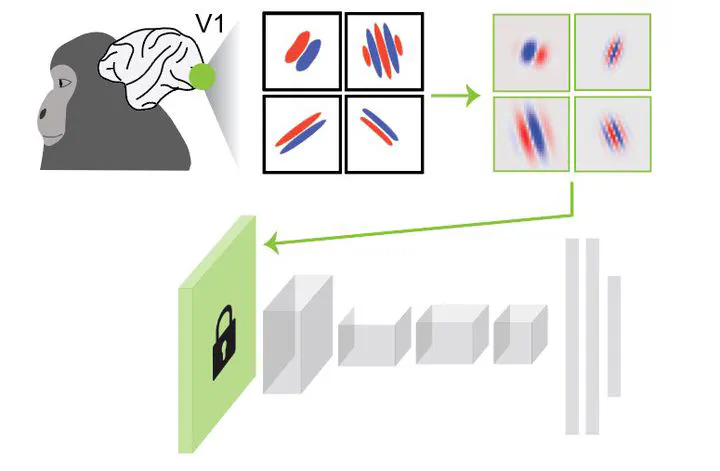
Abstract
Continuously learning new information is a fundamental ability of animals but a challenging problem for conventional deep neural networks (DNNs), which suffer from catastrophic forgetting. Unlike DNNs, whose early layers change depending on training images, the brain’s early visual pathway has innate Gabor-like receptive fields that are stably maintained throughout a lifetime. Here, we demonstrate that fixing early layers of DNNs using Gabor filters, resembling the primary visual cortex (V1) cells’ receptive fields, enables continual learning under dynamic environments. We first showed that networks with fixed Gabor filters maintained the previous performance even when sequentially trained on a completely different image domain, alleviating catastrophic forgetting. Moreover, representation analysis revealed that fixed Gabor filters enabled networks to have similar representations across different domains, which may enable networks to adapt better to continuous learning. Together, Gabor filters in early layers could serve as key architectures for continual learning, highlighting the functional significance of stable early visual pathways in brains.
Type
Publication
bioRxiv